Maximise automation and workflows with MI for greater customer impact
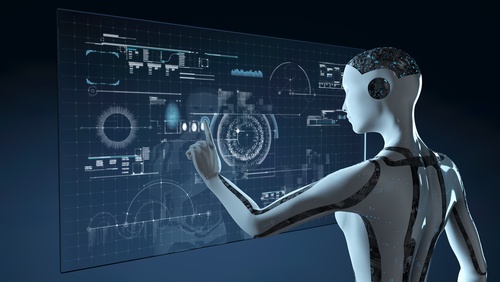
Is Your AI and Automation Strategy Right for You?
In a world where machines can beat humans at Jeopardy! and make complicated medical diagnoses, it's perhaps no surprise that businesses have begun applying automation and artificial intelligence to their internal processes. From sales and marketing to finance and human resources, automation is able to work in a variety of domains in order to unlock countless insights for your organization.
IT research and advisory firm Gartner has called automation "the next frontier for IT." What's more, the company has even predicted that by 2018, more than three million people worldwide will be supervised by "robo-bosses."
Recognizing that an automated sea change is coming in the world of business, many companies have chosen to establish cross-functional teams to manage the automation of their production workflows and data. In most cases, the best choices for processes to automate are those that are repetitive, tedious, rules-based and particularly inefficient to complete by hand.
At least in the beginning, human employees will need to oversee and work in conjunction with these automated processes in order to make sure that they're behaving correctly. This allows you to make smarter decisions that take advantage of the best of what both humans and machines have to offer.
For example, it's easy to imagine a customer service workflow in which users first speak to an AI agent that collects information about the customer's question or issue. In the event that the customer requires advanced assistance, a human customer service representative can take over the call.
However, in many cases, these robotic agents can use AI techniques such as machine learning in order to improve their performance over time without needing to follow the explicit instructions of human monitors. Machine learning refers to the capability of computers to observe patterns in vast quantities of data and then apply those patterns in order to identify objects or classify them into categories. In many cases, computers are able to make predictions with greater accuracy than humans presented with the same data.
For example, credit card companies use machine learning techniques every day to find potentially fraudulent transactions. By analyzing data such as a transaction's date, time, price, location and merchant and learning whether that purchase was authentic, computers can construct their own models of what fraudulent transactions look like and apply them to real-world situations where a customer's behavior appears suspicious or unusual.
Machine learning naturally works best when accompanied by another major technology trend: big data. The more access to relevant training data that computers have, the better they generally perform in practice. Many companies choose to mine their databases for potentially valuable information, check it over manually for any opportunities and then feed it back into the system for automated analysis.
As companies fight to obtain even the slimmest of competitive edges over their rivals, big data has become a big business. IT market intelligence firm IDC has projected that the big data technology and services industry will grow by a blistering 23 percent annually to reach 48.6 billion in 2019.
In the end, the use of technologies like AI, machine learning and data mining all boils down to two things: first, fostering better collaboration and greater efficiency within the organization; and second, offering more pleasant customer experiences. Properly capitalizing on these trends requires companies to understand how they plan to convert their raw data into smart, actionable insights and true business value.